Predictive Maintenance of vehicle doors
Vehicle doors of railway systems are not only mission-critical but also sensible sub-systems. Besides the safety critical function of being shut, when they should be and open when needed, a door system also has to enable fast passenger exchance to prevent unnecessary waiting time at stations.
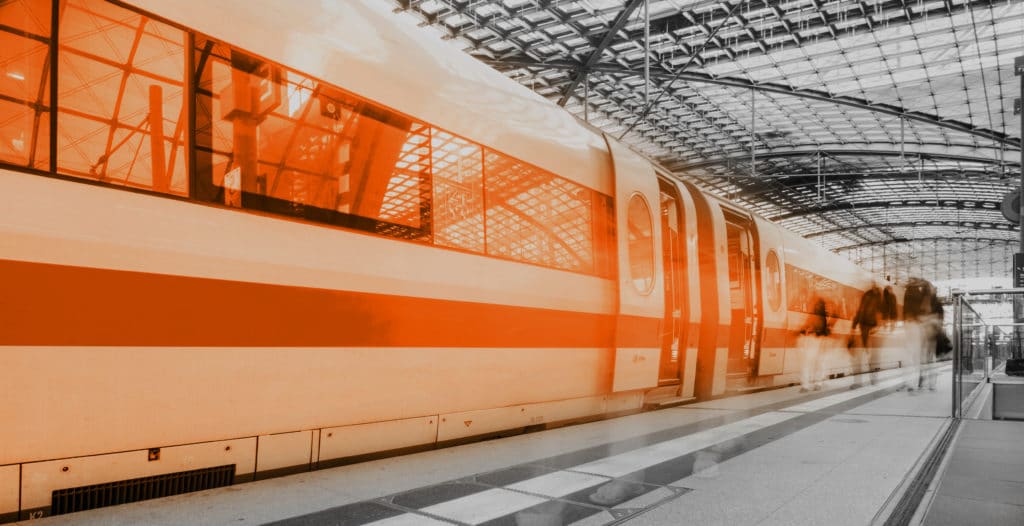
Problem
Condition data of maintenance intensive parts like doors are maybe already created by the manufacturers for own purpose, but it is not granted that those data are accessible to or can be used by the operator.
How it could be
Create, gather, process and analyze diagnosis data of door systems from one to many doors of a complete fleet with only one platform. Reaching from smallest sub-system to board computer, the door maintenance solution can easily being extended to gain value over time. Independent from supplier or vehicle generation.
Why?
Avoid downtime, optimize equipment life cycle, lower your spare costs, save dramatically time in your system management. To make it short: save costs!
The problem: Doors Under Pressure
Doors are mostly mechanical sub systems, often activated, lots of interactions with passengers, exposed to harsh environmental conditions (cold, heat, humidity). Coming with high passenger count, doors often get mechanical stress by being pushed open, being pushed from inside out, when vehicle is full.
Despite all those conditions, doors shall work the whole life cycle of the vehicle, a failing door does not necessarily stop the train, but creates customer dissatisfaction and also potential danger to passengers (think of crowed subway stations – everybody pushes to get in).
Doors consist beside the mechanical parts from 2-3 motors per door to drive the doors in the correct position and electronic control units to (safely) command the door.
Scope of maintenance are the door motors, they face most of the stress (activations, pressure against their drive direction).
Failing motors result in loss of a door function. Doors needs to be closed permanently till next maintenance execution.
Difficult access for high maintenance parts
The door motors and other maintenance intensive parts should be digitalized in order to create condition data of the current health of the motors.
Those condition data are partly already available or created by the door manufacturer for it’s own purpose, but it is neither granted that those data are accessible by the operator (who has the most interest in predictive maintenance) nor that operators gain the right to make use out of them.
The solution: how KYT meets the demands
The KYT portfolio is the ideal platform for
- digitizing the door conditions or access available maintenance data
- collecting data from one to several doors
- combining other metrics like passenger count, daytime, number of activations
- transmitting the condition data to the cloud
- analyzing the data, correlate fleet-wide, derive predictive applications
- deploying and managing your applications in cloud and edge computer
- managing entire fleet remotely from the cloud
By enabling containerized applications running on the edge and in the cloud, the data can be analyzed, and a digital twin of the door can be created.
The condition data from the door(s) can be gathered by several means:
- KYT ModuCop connects to a maintenance interface of the door control unit
- KYT ModuCop connects to the vehicle bus (e.g. MVB), where diagnosis data might already being published
- KYT ModuCop connects own sensors (vibration, temperature, mech. tension) to the sensitive door elements using wired or wireless connection to the edge computer.
After a first phase of data collection over several doors over several vehicles (as more as better) a door behavioral model can be created which – considering historical data and surrounding conditions like temperature, daytime etc – stands for a “good” reference model. Any data from the vehicle can now be compared to the reference model, deviations are identified immediately. Deviations from defined limits are then indicators to a soon failing door. To avoid false positive deviations, data hygiene is mandatory.
With this live indication of the door condition, not only a loss of a door can be predicted, but maintenance activities can be scheduled in advance, meaning a work order for next maintenance execution to check the door, ordering spare parts in time for the workshop, where the train is planned to be within the next maintenance execution.
While getting new data every day, the reference model can be improved continuously which creates an even better interpretation of deviations and therefore a more accurate prediction of failures over time. Feedback from the maintenance workshop also inputs important information to improve the reference model.
Why KYT? These are your Benefits
To bring the advantages to the point: with the use of KYT, you reduce unplanned downtime for higher availability, reduce maintenance duration for higher operational rate, benefit from an optimized use of component life cycle, reduced spare parts and get costly spare parts just in time, which lowers your stocking costs, get a fleet wide transparency of all vehicles and you are able to manage vehicles remotely. All in all – you will save costs.